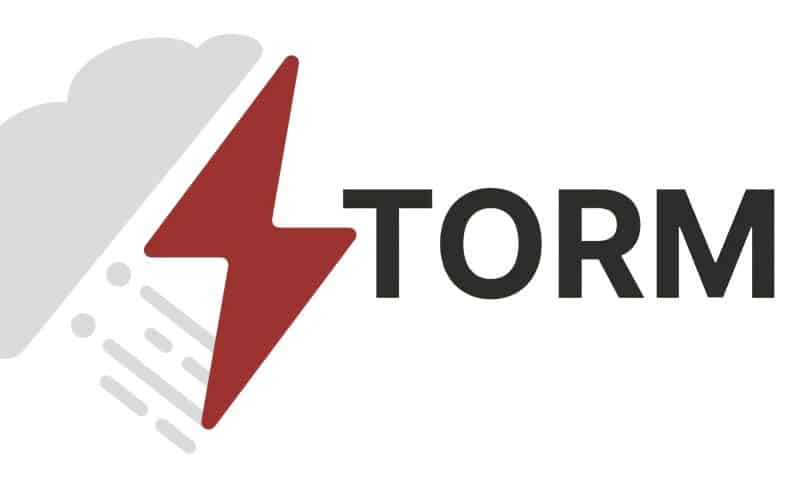
Greenwashing continues to plague the sustainability sector. It completely distorts consumer choices, dilutes authentic efforts, and undermines progress. In response, Stanford University has developed a new AI tool, STORM, designed to expose false environmental claims.
As reported before by us, Greenwashing damages public trust and diverts attention from companies that genuinely invest in sustainability. Once exposed, offenders face reputational loss and a drop in consumer loyalty. Worse, marketing budgets that should amplify verified green achievements often promote empty claims instead.
We tested the new STORM AI tool to see how it exactly works.
How the STORM AI Enters the Picture
Stanford’s AI leverages machine learning, natural language processing (NLP), and deep learning to tackle greenwashing head-on. These tools parse sustainability reports, ads, websites, and public disclosures, flagging vague or exaggerated claims like “eco-friendly,” “green,” or “natural” without supporting data.
By automating this analysis, the AI tool identifies discrepancies between marketing rhetoric and measurable sustainability performance. It can analyze emissions data, energy usage, and even online sentiment around green claims. For example, AI tools can highlight when a company heavily markets its use of recyclable packaging while quietly increasing its overall plastic consumption.
The Stanford system doesn’t just expose misleading language – it scores sustainability claims against industry benchmarks. These scores are cross-validated with independent data sources, offering a high-resolution picture of a company’s environmental footprint.
Such verification pushes companies toward transparency. It also supports regulators and investors by providing consistent, unbiased ESG evaluations – without depending solely on corporate self-reporting.
🚨BREAKING: Stanford University just launched a FREE AI tool for researchers!
— Louis Gleeson (@aigleeson) April 12, 2025
It writes Wikipedia-quality reports with 99% accuracy & citations.
Here’s how to access it for free: pic.twitter.com/5cp2nspDPZ
And for those wonder what the difference is between Stanford’s STORM AI and ChatGPT, know that both cater to different user needs. STORM excels in generating structured, citation-rich content suitable for academic and research purposes. It employs a multi-agent system to simulate diverse perspectives, producing comprehensive reports with transparent sourcing.
However, it may require detailed prompts and lacks conversational flexibility. ChatGPT, on the other hand, offers versatile, conversational interactions, making it ideal for a broad range of tasks, though it may occasionally produce less structured outputs.
In short, if you want structured, source-backed content, then STORM is a good choice. ChatGPT is better suited for interactive, general-purpose use. You can of course combine both to achieve the best result.
Under the Hood: How Stanford’s STORM AI Works
The Stanford STORM AI goes through 7 steps in order to verify data and expose possible false claims:
- Data Ingestion and Preprocessing: The system ingests large volumes of data from corporate sustainability reports, ESG filings, marketing content, environmental audits, and third-party databases. It cleans and standardizes this data to ensure uniformity and remove noise.
- Natural Language Processing (NLP): The NLP engine scans textual content for language patterns associated with greenwashing. It flags unverified claims, ambiguous terms, and marketing buzzwords that lack quantifiable backing. It also parses sentence structure to detect hedging language like “aims to,” “plans to,” or “committed to” without measurable timelines.
- Machine Learning Classification: A supervised ML model classifies statements as verified, unverifiable, misleading, or neutral based on labeled training data. It improves through continuous feedback loops, adapting to new forms of deceptive phrasing.
- Cross-Referencing with Independent Data: The system links textual claims to environmental data sets (e.g., carbon emissions, resource usage, third-party certifications). If a claim lacks matching data, it is flagged as potentially greenwashed.
- ESG Benchmarking: The AI benchmarks companies’ claims against peers and recognized standards (GRI, SASB, CDP, etc.). Deviations from sector norms raise red flags.
- Sentiment and Impact Analysis: The system monitors media and social platforms to correlate public sentiment with corporate disclosures. Mismatches between positive marketing and negative public reaction trigger further scrutiny.
- Scoring and Reporting: Each company receives a transparency score, with contextual explanations. These reports help investors, regulators, and consumers assess whether sustainability claims are grounded in reality or exaggerated for optics.
How to Use Stanford’s STORM AI
Access STORM: Visit storm.genie.stanford.edu and log in using your Google account.
Choose a Mode:
- STORM Mode: Generates comprehensive, citation-backed articles on a given topic.
- Co-STORM Mode: Facilitates interactive, multi-perspective discussions for deeper exploration.
Input Your Topic: Enter the subject matter of your content into the search bar.
Review Generated Content:
- STORM will produce an article with citations for each claim.
- Use the “See BrainSTORMing Process” feature to understand how information was gathered and synthesized.
Compare with Your Content:
- Cross-reference the citations provided by STORM with those in your content.
- Identify any discrepancies or additional sources that could enhance your work.
Refine Your Content:
- Incorporate verified information and citations from STORM to strengthen the credibility of your content.
- Ensure that all claims in your work are supported by reliable sources.
The Future of STORM AI: Verifying User-Provided Content and Links
STORM AI currently excels at generating well-sourced content with transparent citations. Its next evolutionary step hopefully will go far beyond content creation – transforming into a tool that actively verifies the accuracy of user-submitted text and external links.
A verification module should enable users to upload their own articles or paste URLs. STORM could then analyze each claim against reliable data sources. When it detects false, outdated, or misleading information, it should then flag those areas and suggest trusted alternatives.
This upgraded feature should then include:
- Automated Source Cross-Checking: Each claim in the user’s content is matched with data from scientific publications, public databases, and vetted news outlets.
- Link Trust Scoring: Every URL is assessed for credibility and assigned a veracity score, helping users evaluate the strength of their sources.
- Content Consistency Mapping: STORM visualizes the logical flow of the text and points out inconsistencies or disputed sections.
- Real-Time Update Suggestions: If the system detects outdated stats or revised facts, it proposes fresh data points to keep the content current.
STORM AI would then no longer just be a knowledge engine – it would become a digital fact-checker and editorial partner. Whether you write academic papers, corporate reports, or educational material, STORM would then help ensure every word rests on verified ground.
Beyond Greenwashing: The Rise of ‘AI Washing’
AI itself is increasingly subject to similar marketing hype. The term ‘AI washing’ describes the practice of companies overstating or fabricating their use of artificial intelligence to appear more innovative, advanced, or efficient than they actually are. Similar to greenwashing, it involves vague terminology, misleading branding, and empty technological promises.
Companies engaging in AI washing often:
- Claim to use AI without actually implementing it in a meaningful way.
- Use AI-related buzzwords in marketing materials with no technical backing.
- Highlight trivial uses of AI (like basic automation or data sorting) as if they involve advanced machine learning.
- Advertise AI tools that rely heavily on manual input or traditional programming.
Why It Matters: AI washing creates inflated expectations, misleads investors, and distorts competitive landscapes. It also poses risks in regulated sectors like finance, healthcare, and sustainability, where real AI capabilities matter for decision-making and compliance.
Stanford’s AI system can detect AI washing by analyzing technical documentation, patents, deployment logs, and product claims. It checks whether AI-related terms correlate with real technical infrastructure and outcomes. If a company touts AI-driven ESG analysis but cannot provide clear algorithms or datasets, it raises an alert.
Combating AI washing ensures that trust in AI technologies remains intact and that innovation is driven by substance – not spin.
Challenges Remain
Despite the promise, challenges persist:
- Data Privacy: Scrutiny of sensitive internal data requires robust security protocols.
- Bias in AI Models: If trained on skewed data, AI systems can inherit biases.
- Corporate Resistance: Many firms are slow to adopt systems that scrutinize their own marketing.
Sure thing is that we can expect a shift in ESG investing, regulatory enforcement, and consumer decision-making as AI tools like Stanford’s STORM become more accessible. Investors will also gain a clearer picture of impact. And regulatory agencies will have new tools for compliance monitoring. When journalists or even consumers use this or similar tools, they will finally be able to cut through the green noise.
Of course ideally we’d prefer that companies would already have adapted their claims based on STORM. But that is not something we will see happen directly.