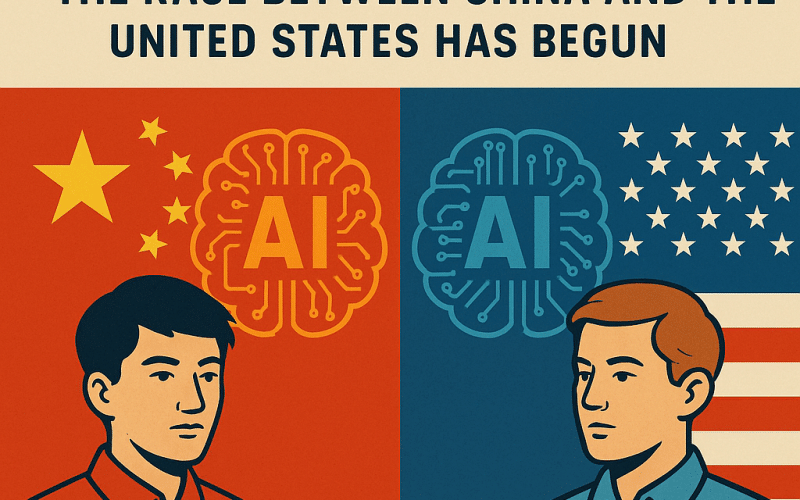
Artificial intelligence (AI) is becoming a foundational technology across industries. However, as we reported before, students graduating now seriously lack AI skills in this transformative market. As a result China announced in 2025 that all students in Beijing would receive mandatory AI training, AI education that is, starting the following academic year. In parallel, the United States initiated its own AI education efforts through a decentralized model.
According to the World Economic Forum, countries that integrate AI into general education early are likely to see significant advantages in workforce readiness. The contrasting strategies however show fundamental differences in governance, educational priorities, and anticipated national outcomes.
Let’s dive into them.
What Is AI Education?
AI education refers to the structured process of teaching students (or any learners) about artificial intelligence technologies, their underlying principles, tools, impacts, and applications. It prepares people to understand, use, create, and critically assess AI systems.
Ideally the precise definition would include these core areas:
1. Technical Foundations
- Machine learning basics (supervised, unsupervised, reinforcement learning)
- Data science principles (data collection, data ethics, data bias)
- Algorithms (neural networks, decision trees, natural language processing, computer vision)
- Programming skills (often in Python, R, or JavaScript relevant to AI models)
- Robotics (optional depending on curriculum)
2. Critical Thinking About AI
- Ethical implications (bias, discrimination, surveillance)
- Social impacts (automation, labor market shifts, misinformation)
- Privacy and security in AI systems
- Regulation and governance (e.g., understanding global AI policies)
3. Applied Skills
- Building simple AI models (e.g., image recognition apps, chatbots)
- Using AI tools (like TensorFlow, PyTorch, or even accessible platforms like Teachable Machine)
- Understanding how AI is deployed in industries like healthcare, finance, education, and agriculture.
4. Future Skills for the Workforce
- Problem-solving with AI tools
- Collaboration with AI systems (“human-in-the-loop” design)
- Creativity in leveraging AI for new applications
In short: AI education is not only coding or programming — it’s a multidimensional literacy combining technical, ethical, societal, and creative dimensions around intelligent systems.
China’s Centralized, Mandatory AI Curriculum
Beijing’s policy requires all students, from primary through secondary school, to take AI courses starting in fall 2025. The program is part of China’s broader “Next Generation Artificial Intelligence Development Plan” (2017).
- Curriculum: Standardized across schools, the program includes core topics such as machine learning fundamentals, data science, and programming skills.
- Teacher Training: Nationwide certification programs, mandatory for all AI instructors, are delivered through centralized summer training initiatives.
- Implementation: Courses are compulsory and integrated within existing STEM curricula.
Example: In a 2024 pilot in Hangzhou, AI modules were introduced to 5th-grade students. Within one year, elective enrollments in advanced AI courses increased by 25% among high school students.
United States’ Decentralized, Voluntary AI Initiatives
The United States launched a federal program in 2024 providing grants to states and districts to incorporate AI education.
- Curriculum: Guided by voluntary frameworks like the AI4K12 guidelines, each state retains autonomy over AI curriculum development.
- Teacher Training: Professional development initiatives such as the NSF-funded AI Institutes support training, but participation is voluntary.
- Implementation: Adoption varies widely by state and district, depending on local priorities and resource availability.
Example: California introduced optional AI modules into its computer science curriculum in 2024. Implementation expanded to 500 schools within a year, though early evaluations revealed wide disparities: some districts built dedicated AI labs, while others offered only basic introductory materials.
Educational Outcomes: A Comparative Analysis
Below is a comparative overview of AI education strategies in China and the United States.
Dimension | China | United States |
---|---|---|
Curriculum Consistency | Uniform and standardized nationwide | Highly variable across states and districts |
Teacher Preparedness | National mandatory certification | Voluntary and inconsistent participation |
Equity of Access | High in urban areas, variable in rural regions | Significant disparities by income and region |
Innovation and Flexibility | Limited within strict national guidelines | High, driven by local experimentation |
Anticipated Early Outcomes | Broad national AI literacy by 2030 | Clustered excellence with widespread gaps |
The Race for Standardized AI Competencies Has Only Begun
China’s strategy is designed to ensure that by 2030, the majority of its workforce will possess standardized AI competencies, aligning with national industrial and economic objectives. This approach minimizes variation across regions but limits local adaptation and curricular innovation.
The United States’ model is producing strong programs in wealthier, better-resourced districts, but large segments of students remain underserved. Without stronger national coordination, the U.S. risks producing elite pockets of AI expertise while leaving the broader workforce underprepared.
By 2030, China is likely to achieve nationwide basic AI literacy in urban regions, directly supporting its broader industrial policies. The United States will likely maintain leadership in AI innovation at the elite level but will face increased socioeconomic divides in technology access and workforce participation.