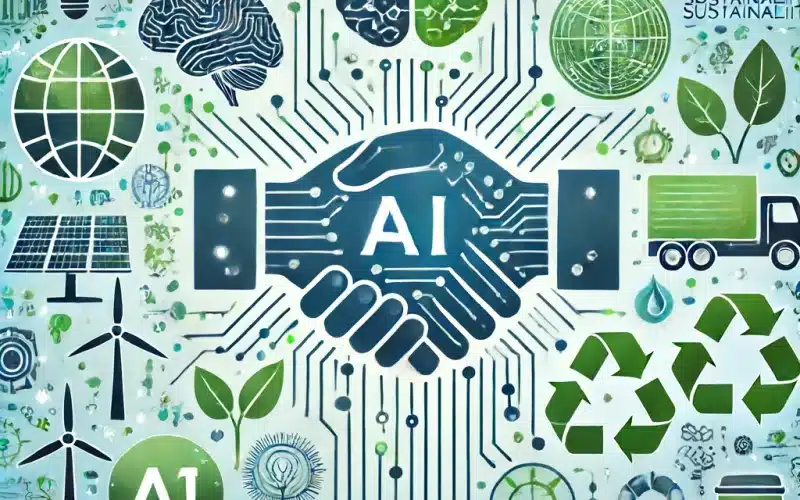
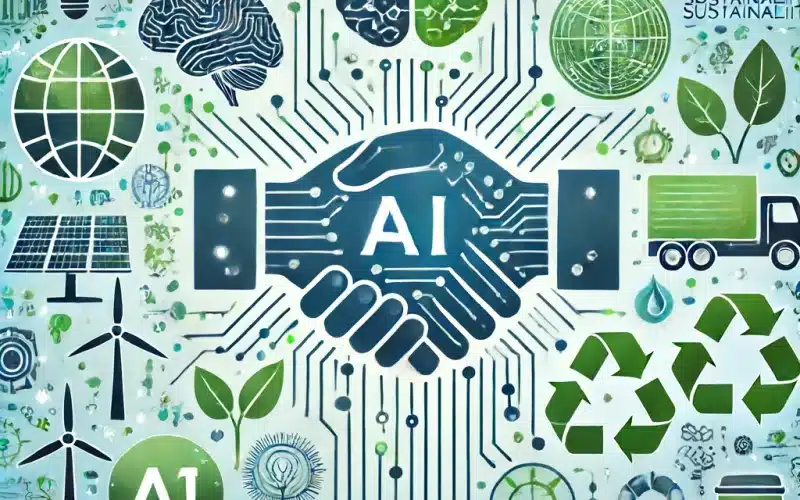
AI and sustainability can indeed be a harmonious pairing if executed well. In the past we already published an article on how AI systems have revolutionized water management and in this article we explore some technical solutions that could largely reduce the energy consumption. But let’s first look at the current energy consumption status of AI.
In the U.S., data center electricity consumption, largely driven by AI, is expected to triple from 2022 levels, reaching up to 390 terawatt-hours by 2030. This increase could account for about 7.5% of the nation’s projected electricity demand. The growing use of AI is also putting a strain on local power grids, causing delays in the planned closure of fossil fuel plants to meet rising energy needs. Globally, AI-related electricity consumption could increase by 85.4 to 134.0 TWh annually by 2027, a figure comparable to the entire electricity consumption of countries like the Netherlands, Argentina, or Sweden.
AI servers generate significant heat and require robust cooling systems. Training AI models involves processing vast datasets, which demands substantial computational power and energy. The storage of these datasets in large data centers further adds to the energy load. Specialized hardware like GPUs and TPUs, used in AI training, are highly energy-intensive as they perform billions of operations per second.
AI applications often rely on continuous learning and model updates, necessitating repeated data processing and retraining to maintain accuracy. The nature of AI, especially deep learning, demands high-performance computing (HPC) environments designed to handle heavy computational loads. Once trained, AI models deployed for inferencing also require substantial computational resources and energy.
8 Strategies to Enhance AI Efficiency and Minimize Environmental Impact
However, several strategies can reconcile AI use in server operations with sustainability, enhancing efficiency while minimizing environmental impact:
- Energy-Efficient Technology: AI servers and data centers should utilize energy-efficient technology and environmentally responsible cooling methods. Modern servers and storage devices designed for sustainability can significantly reduce the carbon footprint. Using PCs and hardware made from sustainable materials, such as recycled plastics and low-carbon aluminum, is crucial. Implementing these methods can reduce energy consumption by up to 30% compared to traditional systems.
- Renewable Energy: Powering data centers with renewable energy sources is essential. Companies like Microsoft and Amazon invest in renewable energy to power their AI operations, reducing reliance on fossil fuels and lowering greenhouse gas emissions. This shift can offset 50-100% of the carbon footprint associated with AI operations.
- Optimized AI Workloads: Right-sizing AI workloads and implementing efficient data center economics can help. Using domain-specific AI models optimized for particular tasks reduces unnecessary computational overhead and energy consumption. This approach can lead to a 20-40% reduction in energy usage.
- Efficient Parameterization and Meta-Learning: Techniques like efficient parameterization and meta-learning can reduce the energy needed for AI model training by up to 50%. These methods optimize computational resources, focusing only on relevant data points and reducing redundancy in model parameters.
- Innovative Cooling Solutions: Advanced cooling methods, such as liquid cooling, can significantly cut down energy and water use. These methods are particularly effective for AI chips, which require substantial cooling to operate efficiently.
- Circular Economy Practices: Emphasizing the refurbishment, reuse, and recycling of AI hardware components can reduce e-waste and keep materials in the circular economy longer. This practice minimizes environmental impact and supports sustainable IT infrastructure.
- AI for Sustainability Solutions: AI can accelerate the development of sustainability solutions, enhancing renewable energy production, optimizing energy storage, reducing food waste, and developing climate-resilient crops. By optimizing processes and making operations more efficient, AI directly contributes to sustainability goalse.
- Monitoring and Reporting: Utilizing AI to monitor and report on sustainability metrics helps organizations track progress and identify areas for improvement. AI-driven analytics provide detailed insights into energy usage, emissions, and overall environmental impact, enabling better decision-makinge.
Sustainability is possible in the AI industry
While AI’s energy consumption presents enough challenges, implementing these strategies can dramatically reduce its environmental impact as shown above. By integrating energy-efficient technology, renewable energy, optimized workloads, and innovative cooling solutions, AI can become a powerful ally in the quest for sustainability. The key lies in balancing technological advancement with responsible environmental stewardship.